The term “artificial intelligence,” or AI, has become a buzzword in recent years. Optimists see AI as the panacea to society’s most fundamental problems, from crime to corruption to inequality, while pessimists fear that AI will overtake human intelligence and crown itself king of the world. Underlying these two seemingly antithetical views is the assumption that AI is better and smarter than humanity and will ultimately replace humanity in making decisions.
It is easy to buy into the hype of omnipotent artificial intelligence these days, as venture capitalists dump billions of dollars into tech start-ups and government technocrats boast of how AI helps them streamline municipal governance. But the hype is just hype: AI is simply not as smart as we think. The true threat of AI to humanity lies not in the power of AI itself but in the ways people are already beginning to use it to chip away at our humanity.
AI outperforms humans, but only in low-level tasks.
Artificial intelligence is a field in computer science that seeks to have computers perform certain tasks by simulating human intelligence. Although the founding fathers of AI in the 1950s and 1960s experimented with manually codifying knowledge into computer systems, most of today’s AI application is carried out via a statistical approach through machine learning, thanks to the proliferation of big data and computational power in recent years. However, today’s AI is still limited to the performance of specialized tasks, such as classifying images, recognizing patterns and generating sentences.
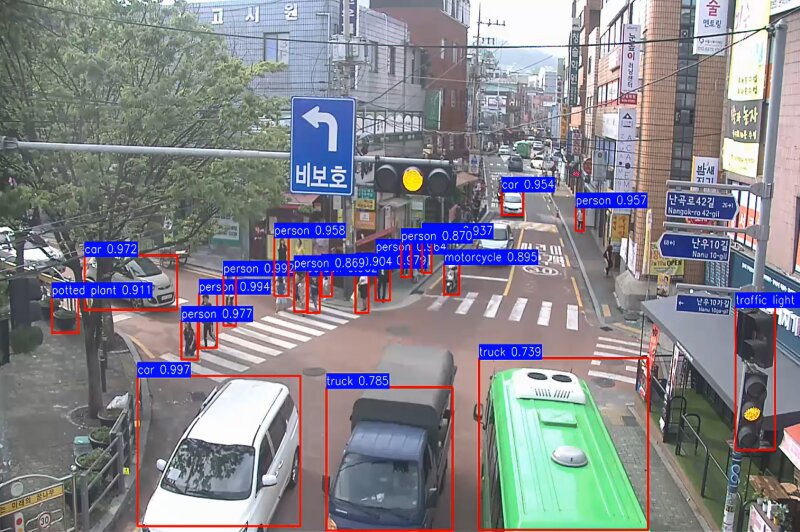
Although a specialized AI might outperform humans in its specific function, it does not understand the logic and principles of its actions. An AI that classifies images, for example, might label images of cats and dogs more accurately than a human, but it never knows how a cat is similar to and different from a dog. Similarly, a natural language processing (NLP) AI can train a model that projects English words onto vectors, but it does not comprehend the etymology and context of each individual word. AI performs tasks mechanically without understanding the content of the tasks, which means that it is certainly not able to outsmart its human masters in a dystopian manner and will not reach such a level for a long time, if ever.
AI does not dehumanize humans — humans do.
AI does not understand humanity, but the epistemological wall between AI and humanity is further complicated by the fact that humans do not understand AI, either. A typical AI model easily contains hundreds of thousands of parameters, whose weights are fine-tuned according to some mathematical principles in order to minimize “loss,” a rough estimate of how wrong the model is. The design of the loss function and its minimization process are often more art than science. We do not know what the weights in the model mean or how the model predicts one result rather than another. Without an explainable framework, decision-making driven by AI is a black box, unaccountable and even inhumane.
This is more than just a theoretical concern. This year in China, local authorities rolled out the so-called “health code,” a QR code assigned to each individual using an AI-powered risk assessment algorithm indicating their risk of contracting and spreading COVID-19. There have been numerous pieces of news coverage about citizens who found their health codes suddenly turning from green (low-risk) to red (high risk) for no reason. They became “digital refugees” as they were immediately banned from entering public venues, including grocery stores, which require green codes. Nobody knows how the risk assessment algorithm works under the hood, yet, in this trying time of coronavirus, it is determining people’s day-to-day lives.
AI applications can intervene in human agency.
Artificial intelligence is also transforming the medical industry. Predictive algorithms are now powering brain-computer interfaces (BCIs) that can read signals from the brain and even write in signals if necessary. For example, a BCI can identify a seizure and act to suppress the symptom, a potentially life-saving application of AI. But BCIs also create problems concerning agency. Who is controlling one’s brain — the user or the machine?
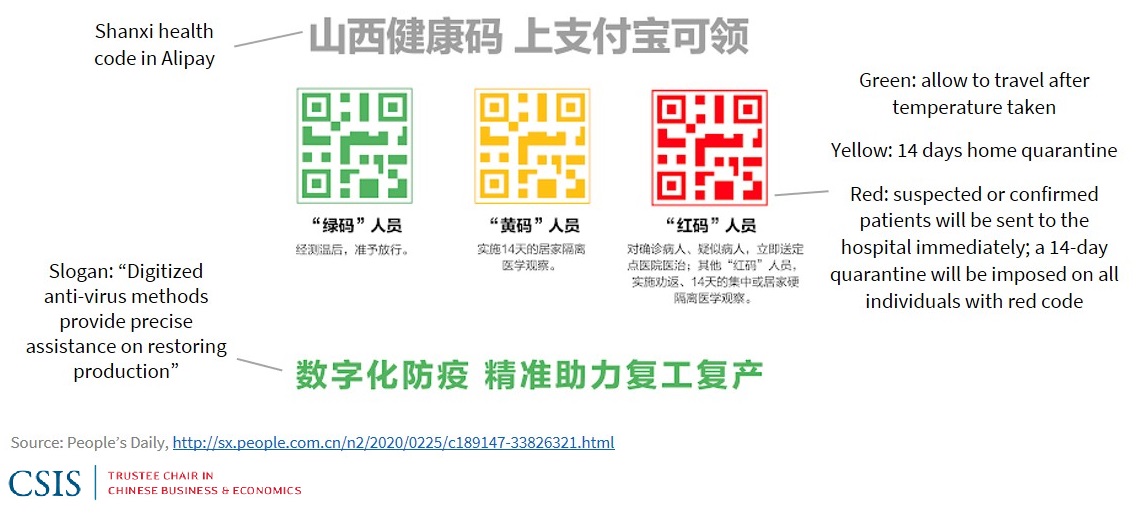
One need not plug their brain into some electronic device to face this issue of agency. The newsfeed of our social medias is constantly using artificial intelligence to push us content based on patterns from our views, likes, moves of the mouse and number of seconds we spend scrolling through a page. We are passive consumers in a deluge of information tailored to our tastes, no longer having to actively reach out to find information — because that information finds us.
AI knows nothing about culture and values.
Feeding an AI system requires data, the representation of information. Some information, such as gender, age and temperature, can be easily coded and quantified. However, there is no way to uniformly quantify complex emotions, beliefs, cultures, norms and values. Because AI systems cannot process these concepts, the best they can do is to seek to maximize benefits and minimize losses for people according to mathematical principles. This utilitarian logic, though, often contravenes what we would consider noble from a moral standpoint — prioritizing the weak over the strong, safeguarding the rights of the minority despite giving up greater overall welfare and seeking truth and justice rather than telling lies.
The fact that AI does not understand culture or values does not imply that AI is value-neutral. Rather, any AI designed by humans is implicitly value-laden. It is consciously or unconsciously imbued with the belief system of its designer. Biases in AI can come from the representativeness of the historical data, the ways in which data scientists clean and interpret the data, which categorizing buckets the model is designed to output, the choice of loss function and other design features. A more aggressive company culture, for example, might favor maximizing recall in AI, or the proportion of positives identified as positive, while a more prudent culture would encourage maximizing precision, the proportion of labelled positives that are actually positive. While such a distinction might seem trivial, in a medical setting, it can become an issue of life and death: do we try to distribute as much of a treatment as possible despite its side effects, or do we act more prudently to limit the distribution of the treatment to minimize side effects, even if many people will never get the treatment? Within a single AI model, these two goals can never be achieved simultaneously because they are mathematically opposed to each other. People have to make a choice when designing an AI system, and the choice they make will inevitably reflect the values of the designers.
Take responsibility, now.
AI may or may not outsmart human beings one day — we simply do not know. What we do know is that AI is already changing power dynamics and interpersonal relations today. Government institutions and corporations run the risk of treating atomized individuals as miniscule data points to be aggregated and tapped by AI programs, devoid of personal idiosyncrasies, specialized needs, or unconditional moral worth. This dehumanization is further amplified by the winner-takes-all logic of AI platform economies that creates mighty monopolies, resulting in a situation in which even the smallest decisions made by these companies have the power to erode human agency and autonomy. In order to mitigate the side effects of AI applications, academia, civil society, regulators and corporations must join forces in ensuring that human-centric AI will empower humanity and make our world a better place.
Featured image source: Odyssey
Comments are closed.